If you need assistance with Fourier Analysis, you've come to the right place. Whether you're looking for a deeper understanding of its principles or practical applications, our blog is here to help. We offer clear explanations, step-by-step guides, and insightful examples to support your journey into the fascinating world of Fourier Analysis. If you want more assistance submit your assignment at TakeMyClassCourse.com
The Fourier transform is a potent mathematical tool that revolutionizes the analysis and manipulation of functions and signals. It is named after the renowned mathematician Jean-Baptiste Joseph Fourier. The Fourier transform reveals the complex interrelationship between various frequency components by representing a function or signal in the frequency domain, offering insightful information about their strengths, phases, and interaction. We will delve into the complexities of the Fourier transform in this article and examine its ten key points in depth, illuminating its uses and importance in various fields.
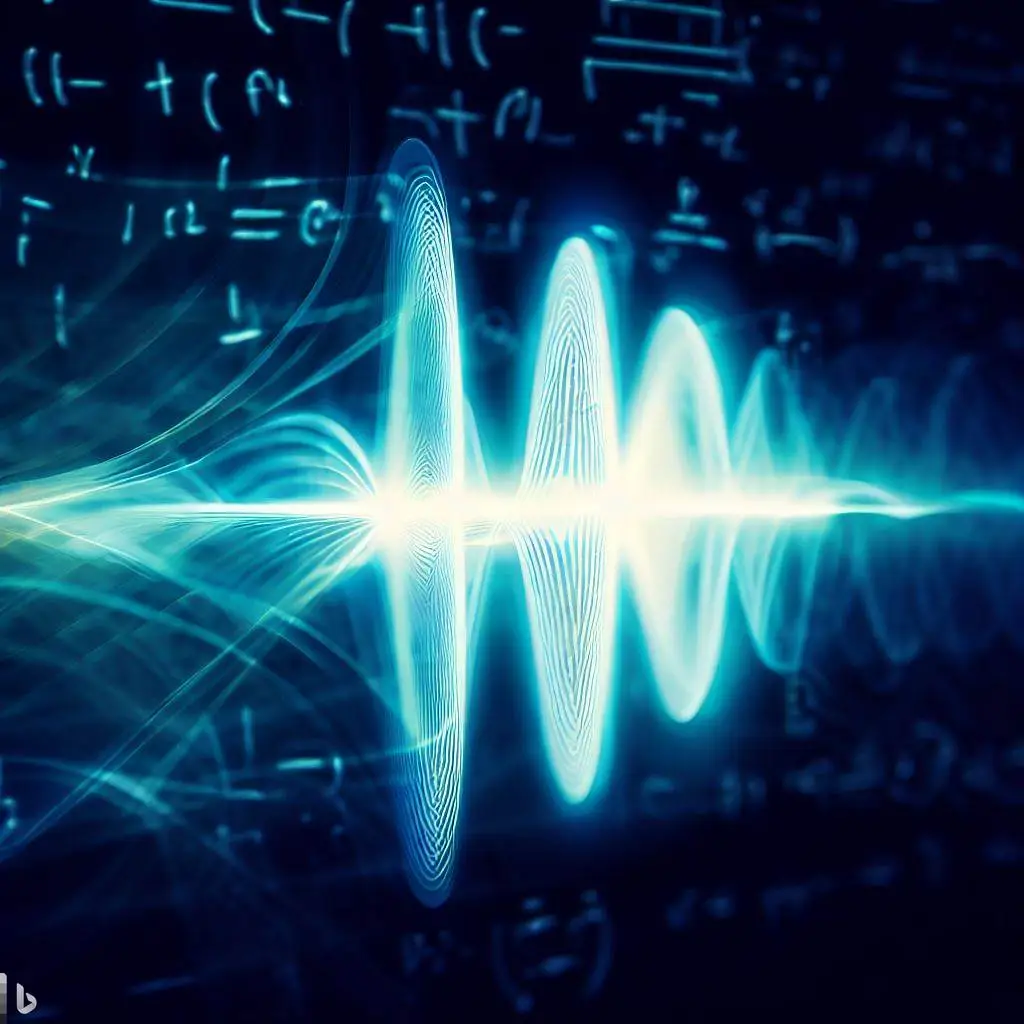
Frequency Domain Representation:
A mathematical method called the Fourier transform enables us to represent a function or signal in the frequency domain. By expressing the function as the sum of sinusoidal components with various frequencies, amplitudes, and phases, it offers a fresh viewpoint on the function. The distribution of frequencies in the signal and their individual contributions are shown by this representation.
Analysis of Frequency:
We can examine the frequency content of a function or signal using the Fourier transform. We can assess the potency and significance of each frequency by breaking the function down into its individual frequency components. The signal's dominant frequencies, harmonic relationships, and periodicity can all be found using this analysis.
Fourier transform is a particularly effective tool for analyzing periodic functions. It enables us to divide a periodic function into several sine and cosine functions, each of which corresponds to a different frequency. The fundamental frequency, which is the primary frequency component, and its harmonics, which are integer multiples of the fundamental frequency, are explained by this representation.
Fourier Transform:
The Fourier transform can be divided into two types: continuous and discrete. When a signal is defined over a continuous period of time, it is subject to the continuous Fourier transform. We can represent functions with infinite time duration using the continuous Fourier transform equation, which involves an integral. On the other hand, discrete-time signals that are sampled at predetermined intervals are used with the discrete Fourier transform (DFT). The DFT is appropriate for digital signals and discrete datasets because it transforms a series of discrete samples into its frequency domain representation.
Fast Fourier Transform:
The discrete Fourier transform (DFT) can be computed quickly using the Fast Fourier Transform (FFT). It significantly lowers the Fourier transform's computational complexity from O(n2) to O(n log n), where n is the total number of data points. The FFT algorithm is widely used in real-world applications like digital signal processing, telecommunications, and data analysis because it takes advantage of the symmetries and properties of complex numbers to speed up computation.
Due to the use of complex exponentials in the representation of frequency components, the Fourier transform involves complex numbers. Complex numbers can be used to represent frequency components' magnitude and phase because they have both real and imaginary parts. The phase shift is represented by the imaginary portion of the Fourier transform, while the magnitude or amplitude of each frequency component is represented by the real portion.
Inverse Fourier Transform:
The inverse Fourier transform is the inverse of the Fourier transform. It enables us to reconstruct the original function from its frequency domain representation in the time (or space) domain. Integrating the Fourier coefficients and complex exponentials results in the equation for the inverse Fourier transform. We can return the frequency domain representation to the original time domain representation by using the inverse Fourier transform, allowing for analysis and manipulation across both domains.
The Fourier transform demonstrates a potent characteristic known as the convolution theorem. This theorem states that the pointwise multiplication of two functions' Fourier transforms in the frequency domain corresponds to the convolution of those two functions in the time domain. Convolution analysis and manipulation, which are crucial steps in signal processing, filtering, and image processing, are made easier by this property. By avoiding the computational complexity of time-domain convolution, the convolution theorem enables us to perform convolutions more effectively in the frequency domain.
Applications in Signal Processing:
Fourier transform is crucial in applications for signal processing. Signals can be analyzed spectrally, have unwanted noise or interference removed, have filtering operations applied, have data compressed, and have modulation or demodulation performed by converting them into the frequency domain. In many different applications where signal manipulation is necessary, including telecommunications, radar systems, biomedical signal analysis, and audio and video processing, Fourier transform-based techniques are widely used.
Widely Used:
The Fourier transform is used for more than just signal processing. It has applications in many areas, including image processing (for image enhancement, compression, and analysis), audio analysis (for music synthesis, sound recognition, and audio effects), quantum mechanics (for wavefunction analysis and quantum algorithms), medical imaging (for MRI and CT scan reconstruction), communication systems (for modulation, demodulation, and channel estimation), and many others where the frequency characteristics of functions and signals are important. The Fourier transform is a fundamental tool for comprehending and modifying signals and data across numerous disciplines due to its adaptability.
Lets break down the equation for fourier transform:
F(ω) = ∫[f(t) * e^(-iωt)] dt
Where:
- F(ω) represents the transformed function in the frequency domain.
- f(t) is the original function in the time (or space) domain.
- ω is the angular frequency.
A Challenging Example:
To fully grasp the intricacies of Fourier analysis, let's consider a longer and tougher example involving a non-periodic function. Suppose we have the following function:
f(t) = e^(-|t|)
This function represents a symmetric exponential decay, which has applications in various fields such as physics and finance. Our objective is to analyze this function using Fourier techniques.
Solution:
Step 1: Begin by writing down the Fourier integral equation for the given function:
F(ω) = ∫[f(t) * e^(-iωt)] dt
In our case, f(t) = e^(-|t|). We need to find the Fourier transform F(ω) of this function.
Step 2: Substitute the expression for f(t) into the Fourier integral equation:
F(ω) = ∫[(e^(-|t|)) * e^(-iωt)] dt
Step 3: Simplify the equation by multiplying the exponentials:
F(ω) = ∫[e^(-|t| - iωt)] dt
Step 4: Split the integral into two parts:
F(ω) = ∫[e^(-|t|)] * ∫[e^(-iωt)] dt
The first integral represents the Fourier transform of the absolute value function e^(-|t|), while the second integral represents the Fourier transform of the complex exponential function e^(-iωt).
Step 5: Solve the first integral:
∫[e^(-|t|)] dt
This integral can be challenging to solve analytically due to the absolute value function. However, we can use symmetry properties to simplify the integral. Since e^(-|t|) is an even function, we can rewrite the integral as:
2 * ∫[e^(-t)] dt, for t >= 0
The integral of e^(-t) is a well-known function and can be easily solved:
2 * ∫[e^(-t)] dt = -2 * e^(-t)
Applying the limits, we have:
2 * ∫[e^(-t)] dt = -2 * (e^(-t) - e^0) = -2 * (e^(-t) - 1), for t >= 0
Similarly, for t < 0, the integral is:
2 * ∫[e^(-t)] dt = -2 * ∫[e^t] dt = -2 * (e^t)
Combining both cases, we have:
∫[e^(-|t|)] dt = -2 * (e^(-|t|) - 1)
Step 6: Solve the second integral:
∫[e^(-iωt)] dt
The second integral represents the Fourier transform of the complex exponential function e^(-iωt). This integral is well-known and has a simple solution:
∫[e^(-iωt)] dt = (-i/ω) * e^(-iωt)
Step 7: Substitute the results of the integrals into the Fourier integral equation:
F(ω) = (-2) * ∫[e^(-|t|)] * [(-i/ω) * e^(-iωt)] dt
F(ω) = (2i/ω) * ∫[e^(-|t|)] * e^(-iωt) dt
Step 8: Simplify the expression:
F(ω) = (2i/ω) * ∫[e^((-|t|) - iωt)] dt
Step 9: Perform the integration:
F(ω) = (2i/ω) * ∫[e^(-t - iωt)] dt, for t >= 0
F(ω) = (2i/ω) * ∫[e^(-t + iωt)] dt, for t < 0
Both integrals can be solved by using the properties of the complex exponential function. Applying the limits, we obtain:
F(ω) = (2i/ω) * (1/(1 - iω)), for t >= 0
F(ω) = (2i/ω) * (1/(1 + iω)), for t < 0
Step 10: Simplify further:
To simplify the expression, we can multiply the numerator and denominator by the complex conjugate of the denominator:
F(ω) = (2i/ω) * [(1/(1 - iω)) * (1 + iω)/(1 + iω)], for t >= 0
F(ω) = (2i/ω) * [(1/(1 + iω)) * (1 - iω)/(1 - iω)], for t < 0
Expanding and simplifying, we get:
F(ω) = (2i/ω) * [(1 + iω)/(1 + ω^2)], for t >= 0
F(ω) = (2i/ω) * [(1 - iω)/(1 + ω^2)], for t < 0
Thus, we have obtained the Fourier transform F(ω) of the function f(t).
Conclusion:
By breaking complex functions down into their simpler harmonic components, Fourier analysis is a powerful mathematical tool that enables us to comprehend and analyze them. We used the steps of Fourier analysis in our challenging example to obtain an expression for the Fourier transform of the non-periodic function. We came up with a solution for the Fourier transform by making use of symmetry properties and using methods for evaluating integrals.
Mathematicians, scientists, and engineers can discover the hidden properties and spectral characteristics of complex functions by mastering Fourier analysis and using cutting-edge techniques. Numerous fields find uses for Fourier analysis, which advances science, technology, and our comprehension of the world.